19. Biology – phylogeny
[status: written, but incomplete]
19.1. Motivation, prerequisites, plan
19.1.1. Motivation
One of the most important areas of research in biology is that of phylogenetic analysis. This collection of techniques allows us to build an evolutionary tree showing how various species are related.
This type of analysis can also be used in other areas, such as tracing the origin of human spoken languages.
I find phylogenetic analysis to be fascinating because it gives us a sort of “webcam of the gods”, a view of the past (which we cannot see) which brought about the present state of things.
19.1.2. Prerequisites
The 10-hour “serious programming” course.
The “Data files and first plots” mini-course in Section 2
19.1.3. Plan
We will start by looking at a video tutorial of how to build a simple phylogenetic tree by hand. Then we will learn how to use biopython and ete3 packages to construct and visualize trees on that simple problem.
Then we discuss further projects in which we look for data sets to work with, including sets from our own genetic algorithm runs (where we also know the real evolutionary history), human languages (where we do not know the real history), and computer programming languages (where we should know most of the real history).
https://cnx.org/contents/24nI-KJ8@24.18:EmlvXoDL@7/Taxonomy-and-phylogeny
19.2. Start with a video and then make a simple table
Start with this Khan Academy tutorial on phylogenetic trees
Then we take their table of traits. Start with the empty table:
Species |
Feathers |
Fur |
Lungs |
Gizzard |
Jaws |
---|---|---|---|---|---|
Lamprey |
|||||
Antelope |
|||||
Sea Bass |
|||||
Bald Eagle |
|||||
Alligator |
and write it on the board. Then fill out the tree on the board with the class. You can discuss what all these animals are, and look them up if necessary.
The table will end up looking like this:
Species |
Feathers |
Fur |
Lungs |
Gizzard |
Jaws |
---|---|---|---|---|---|
Lamprey |
no |
no |
no |
no |
no |
Antelope |
no |
yes |
yes |
no |
yes |
Sea Bass |
no |
no |
no |
no |
yes |
Bald Eagle |
yes |
no |
yes |
no |
yes |
Alligator |
no |
no |
yes |
no |
yes |
Then use the principle of parsimony to create the phylogenetic tree, following the guidelines in the tutorial. The result should look like what you see in Figure 19.2.1.
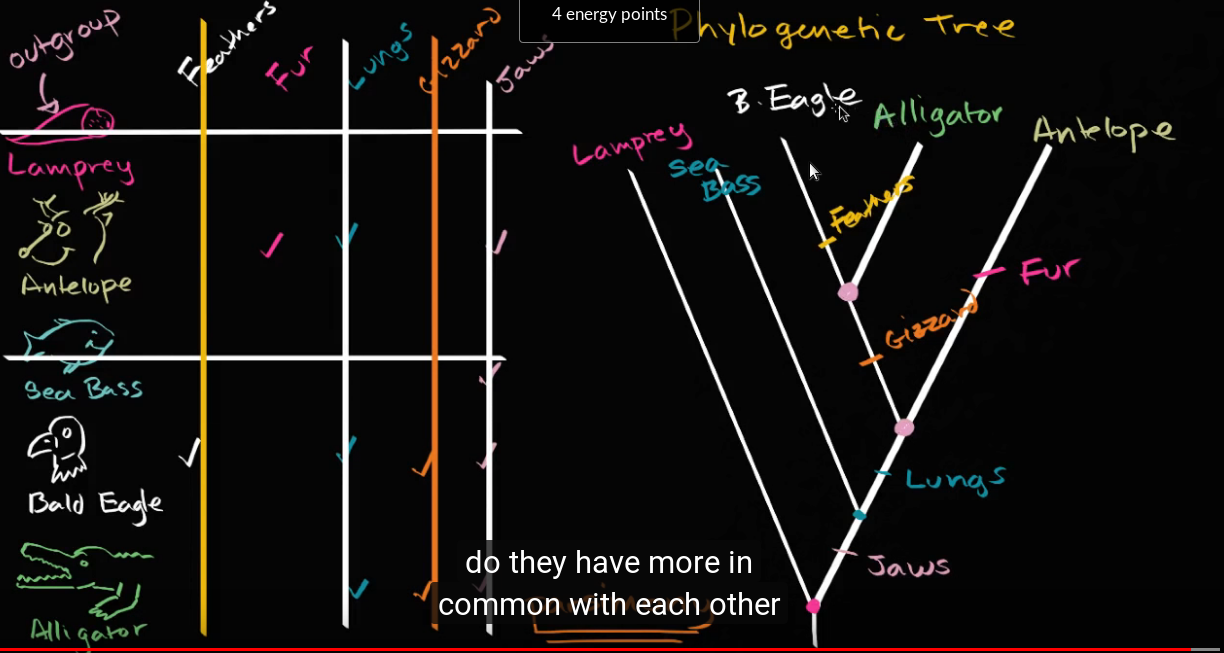
Figure 19.2.1 The resulting tree from the Khan Academy video example.
Discuss the meaning of parsimony as seen in this example. Connect it to Ockham’s razor.
19.3. Terminology
Clades, taxa, species, genotype, phenotype, …
The tree of life
Figure 19.3.1 Hillis’s tree of life based on completely sequenced genomes (from the Wikipedia image)
19.4. NEW - Installing necessary packages
sudo apt install python3-biopython python3-matplotlib
19.5. NEW - first steps with biopython
Tutorials are at:
https://taylor-lindsay.github.io/phylogenetics/
and
http://biopython.org/DIST/docs/tutorial/Tutorial.html
Data from opentreeoflife at:
https://tree.opentreeoflife.org/
I tied Streptococcus_mitis_NCTC_12261_ott725 at:
https://tree.opentreeoflife.org/opentree/argus/ottol@175918/Streptococcus
and downloaded the Newick format of the streptococcus subtree at:
https://tree.opentreeoflife.org/opentree/default/download_subtree/ottol-id/175918/Streptococcus
with:
wget --output-document subtree-ottol-175918-Streptococcus.tre https://tree.opentreeoflife.org/opentree/default/download_subtree/ottol-id/175918/Streptococcus
from io import StringIO
from Bio import Phylo
from Bio.Phylo.TreeConstruction import DistanceCalculator
t = Phylo.read(StringIO("((a,b),c);" ), format="newick")
Phylo.draw(t)
import os
import matplotlib
import matplotlib.pyplot as plt
from Bio import Phylo
from Bio.Phylo.TreeConstruction import DistanceCalculator
os.system('wget https://api.opentreeoflife.org/v3/study/ot_2221.tre')
tree = Phylo.read("ot_2221.tre", "newick")
Phylo.draw(tree)
Phylo.draw_ascii(tree)
os.system('wget --output-document subtree-ottol-175918-Streptococcus.tre https://tree.opentreeoflife.org/opentree/default/download_subtree/ottol-id/175918/Streptococcus')
tree = Phylo.read("subtree-ottol-175918-Streptococcus.tre", "newick")
Phylo.draw(tree)
19.6. OLD - Installing necessary packages
Follow the instructions at http://etetoolkit.org/download/
# Install Minconda (you can ignore this step if you already have Anaconda/Miniconda)
wget http://repo.continuum.io/miniconda/Miniconda3-latest-Linux-x86_64.sh -O Miniconda3-latest-Linux-x86_64.sh
bash Miniconda3-latest-Linux-x86_64.sh -b -p ~/anaconda_ete/
export PATH=~/anaconda_ete/bin:$PATH;
# Install ETE
conda install -c etetoolkit ete3 ete_toolchain
# Check installation
ete3 build check
Now to test it run this simple program. You can even paste it into the python3 interpreter.
from ete3 import Tree
t = Tree( "((a,b),c);" )
t.render("mytree.png", w=183, units="mm")
19.7. Preparing a tree by hand
Now let us prepare a tree where we input it ourselves. The format is
like what we saw in the example above: the tree is made with the call
Tree( "((a,b),c);" )
But we will make a slightly more interesting tree, the one we worked out in Section 19.2. To do so enter the program in Listing 19.7.1.
#! /usr/bin/env python3
from ete3 import Tree
out_fbase = 'tree-sample-animals'
# t = Tree( "((a,b),c);" )
t = Tree( "((((Eagle,Alligator),Antelope),Sea Bass),Lamprey);" )
for format in ('png', 'svg'):
out_fname = out_fbase + '.' + format
print('writing output to', out_fname)
t.render(out_fname, w=183, units="mm")
You can adjust the look of this tree. See the discussion in:
http://etetoolkit.org/docs/3.0/tutorial/tutorial_drawing.html
we can go through that and adjust our styles a bit and see how our tree looks.
19.8. Inferring a tree
The problem with the program in Listing 19.7.1 is that it prepares the tree, which you can view with your favorite PNG or SVG file viewer. But it does not find the tree. That is our next goal.
So we want to find the most likely evolutionary tree that would yield the result we see in the Table 19.2.2. This process is called inferring the phylogenetic tree from the table of characteristics.
19.8.1. An example input file provided by ete3
Following the ete cookbook at http://etetoolkit.org/cookbook/ete_build_basics.ipynb
let us try:
# find some place to download NUP62.aa.fa
$ mkdir phylo
$ cd phylo
$ locate NUP62.aa.fa
/home/markgalassi/anaconda_ete/lib/python3.6/site-packages/ete3/test/test_ete_build/NUP62.aa.fa
/home/markgalassi/anaconda_ete/pkgs/ete3-3.1.1-pyhf5214e1_0/site-packages/ete3/test/test_ete_build/NUP62.aa.fa
$ cp /home/markgalassi/anaconda_ete/lib/python3.6/site-packages/ete3/test/test_ete_build/NUP62.aa.fa ./
$ cat NUP62.aa.fa | head -n15
$ ete3 build -w standard_fasttree -a NUP62.aa.fa -o NUP62_tree/ --clearall
$ ls NUP62_tree/ -ltr
$ geeqie NUP62_tree/clustalo_default-none-none-fasttree_full &
Adapting our table to the .fa
file format, we need a name for each
organism, and an encoding for the traits. The ete3 team’s example has
this line, for example:
>Phy004Z0OU_MELUD
MSQFSFGTGGGFTLGTSGTAASTAATGFSFSSPAGSGGFGLGSAAPAAGSSSQSSGLFSF
SRPAATAAQPGGFSFGTAGTSSAAPAASVFQLGANAPKLSFGSSSATPATGITGSFTFGS
SAPTSAPSSQAAAPGFVFGSAGTSSTAQAGTTAGFTFSSGTTTQAGAGSLSMGAAVPQTA
PTGLSFGAAPAAAATSAATLGAATQPAAPFSLGGQSTATATVSTSTSSGPALSFGAKLGV
TSTSAATASTSTTSVLGSTGPTLFASVASSAAPASSTTTGLSLGAPSTGTASLGTLGFGL
KAPGTTSAATTSTATGTTTASGFALNLKPLTTTGATGAVTSTAAITTTTSTSAPPVMTYA
QLESLINKWSLELEDQEKHFLHQATQVNAWDQTLIENGEKITSLHREVEKVKLDQKRLDQ
ELDFILSQQKELEDLLTPLEESVKEQSGTIYLQHADEEREKTYKLAENIDAQLKRMAQDL
KDITEHLNTSRGPADTSDPLQQICKILNAHMDSLQWIDQNSAVLQRKVEEVTKVCESRRK
EQERSFRITFD
and we could have something like:
>Lamprey
NNNNN
>Antelope
NYYNY
>Sea_Bass
NNNNY
>Bald_Eagle
YNYNY
>Alligator
NNYNY
Put this information into a file called simple-animals.fa
and use
the ete3 build
command to infer a phylogenetic tree for it:
ete3 build -w standard_fasttree -a simple-animals.fa -o simple-animals_tree/ --clearall
This program will put graphical output files in the directory
simple-animals_tree/clustalo_default-none-none-fasttree_full/
and
you can view the .png, .svg and .pdf files there.
The output shows the same family links that we obtained by hand, but the tree looks different because the root is placed differently. FIXME: must find the correct invocation of ete3 to root the tree parsimoniously.
You can also view this tree as ascii with:
ete3 view -t simple-animals_tree/clustalo_default-none-none-fasttree_full/simple-animals.fa.final_tree.nw
We can process it further with a few python instructions:
from ete3 import PhyloTree
tree = PhyloTree("simple-animals_tree/clustalo_default-none-none-fasttree_full/simple-animals.fa.final_tree.nw")
tree.link_to_alignment("simple-animals_tree/clustalo_default-none-none-fasttree_full/simple-animals.fa.final_tree.used_alg.fa")
tree.set_outgroup('Lamprey')
tree.render("%%inline")
tree.render("simple-animals-rooted-outgroup.svg", w=183, units="mm")
tree.render("simple-animals-rooted-outgroup.png", w=183, units="mm")
print(tree)
This will save svg and png formatted views of the tree, as well as showing you an ascii representation.
The documentation on ete3 show a dizzying variety of tree styles. The key is to define a tree style using the Python TreeStyle class, and then use it as a parameter to how we visualize our tree.
Here are a couple of of examples, continuing from the previous code. The first, taken from the ETE tutorial, shows a circular tree in 180 degrees.
# [this uses the tree built in the previous code block]
from ete3 import Tree, TreeStyle
ts = TreeStyle()
ts.show_leaf_name = True
ts.mode = "c"
ts.arc_start = -180 # 0 degrees = 3 o'clock
ts.arc_span = 180
tree.render("simple-animals-circular.svg", tree_style=ts)
tree.render("simple-animals-circular.png", tree_style=ts)
tree.show(tree_style=ts)
## note that instead of tree.show(), which opens a live tree
## browser, you could use tree.render() to save it to a file
Another example shows our tree as a bubble tree map:
19.9. Other sequence analysis resources
Berkeley evolution course. 7 organisms and 7 features: https://evolution.berkeley.edu/evolibrary/article/phylogenetics_07
Cute with ladybugs, but just 6 elements and 7 features: https://bioenv.gu.se/digitalAssets/1580/1580956_fyltreeeng.pdf
Another video giving step-by-step for building a tree by hand: https://www.youtube.com/watch?v=09eD4A_HxVQ
19.10. Linguistics datasets
First discuss the issues of generating a string representation of language features. One example of the issues involved is given in this article:
https://brill.com/view/journals/ldc/3/2/article-p245_4.xml?lang=en
where they discuss how to align the English “horn” with the latin “kornu”. This then allows you to define a “genetic distance” between the same word in two different languages. One such measure is the “Levenshtein normalized distance” (LDN), which takes values between 0 and 1.
This can then be used with the ASJP (Automated Similarity Judgement Program) https://en.wikipedia.org/wiki/Automated_Similarity_Judgment_Program database which is based on a word list. The database is at https://asjp.clld.org/
Download the database from
https://asjp.clld.org/download
and look at the listss18.txt file and see how languages we know (English, Italian, Spanish, Russian) are represented.
Look at the results in WorldLanguageTree001.pdf
One oft-used list is the the Swadesh list https://en.wikipedia.org/wiki/Swadesh_list which has 100 terms. Some of these are “I”, “you”, “we”, “this”, “that”, “person”, “fish”, “dog”, “foot”, “hand”, “sun”, “mountain”, various basic colors, and so forth. There is also an abbreviated 35-word list. The ASJP uses a 40-word list, similar to the Swadesh list.
Each part of a word gets an ASJP code and an IPA (International Phonetic Alphabet) designation of how it’s pronounced.
19.10.1. lingpy.org
Go through the tutorial, starting at:
http://www.lingpy.org/tutorial/index.html
install with
pip3 install lingpy
then simple examples at:
http://www.lingpy.org/examples.html
then the workflow tutorial at:
http://www.lingpy.org/tutorial/workflow.html
and the cookbook at:
19.10.2. elinguistics.com
Overall language evolutionary tree: http://www.elinguistics.net/Language_Evolutionary_Tree.html You can follow the links to some detailed discussion of timelines at http://www.elinguistics.net/Language_Timelines.html as well as in-depth discussion of encoding and language comparison. In particular you will find various sounds for key encoding words at http://www.elinguistics.net/Compare_Languages.aspx?Language1=English&Language2=German&Order=Details
The choice of “basis words” to use as “genetic markers” is described at http://www.elinguistics.net/Lexical_comparison.html and the continuing pages http://www.elinguistics.net/Sound_Correspondence.html and back to the example of English to German at http://www.elinguistics.net/Compare_Languages.aspx?Language1=English&Language2=German&Order=Details
19.10.3. Others
https://en.wikipedia.org/wiki/Tree_model#Perfect_phylogenies
https://en.wikipedia.org/wiki/Tree_model
https://en.wikipedia.org/wiki/Language_family
https://en.wikipedia.org/wiki/Tree_model#CITEREFNakhleh2005
https://www.theguardian.com/education/gallery/2015/jan/23/a-language-family-tree-in-pictures
https://www.ncbi.nlm.nih.gov/pmc/articles/PMC3049109/
https://glottolog.org/resource/languoid/id/stan1293
https://glottolog.org/glottolog/family
https://www.ethnologue.com/browse/families
https://glottolog.org/resource/languoid/id/macr1271
https://science.sciencemag.org/content/323/5913/479
Look at the PDF for this paper on phylogeny of polynesian languages:
And this one with very nice-looking pictures of Japonic language evolution and some discussion of word differentiation.
https://royalsocietypublishing.org/doi/full/10.1098/rspb.2011.0518
Natural language processing with Python and NLTK:
19.11. Evolution of programming languages
https://www.i-programmer.info/news/98-languages/8809-the-evolution-of-programming-languages.html
https://royalsocietypublishing.org/doi/full/10.1098/rsif.2015.0249